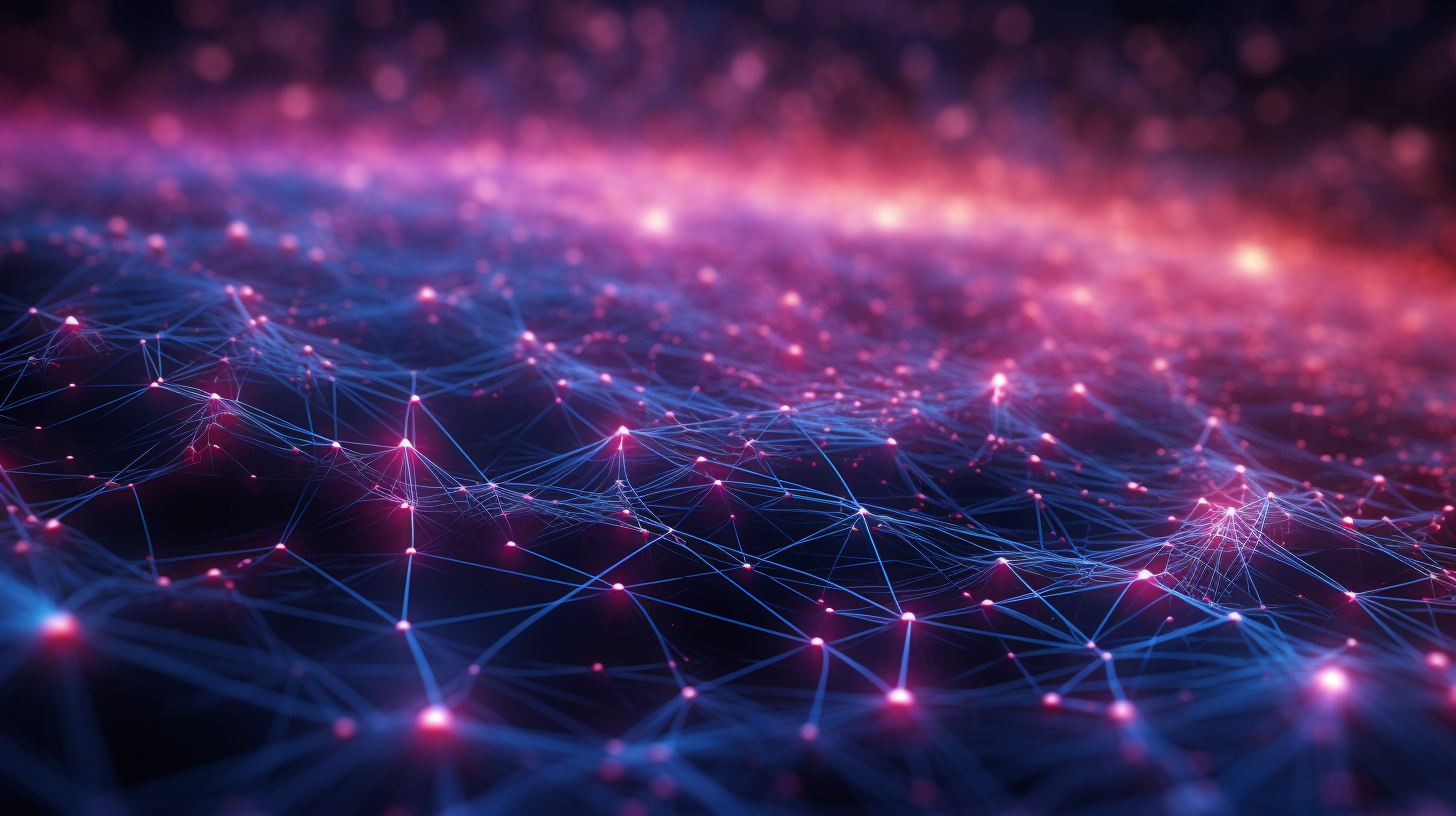
Gene therapy has the potential to revolutionize medicine by addressing genetic disorders at their root, offering the prospect of one-time, targeted, and personalized treatments that could significantly improve the lives of those with genetic diseases.
Adeno-associated virus (AAV) is a small, non-pathogenic virus commonly used in gene therapy because it can achieve long-term transgene gene expression without integrating into the host genome. Its ability to infect both dividing and non-dividing cells, high transduction efficiency to target cells, and minimal risk of causing disease make AAV a preferred vector for gene therapy applications.1
Yet, designing AAV constructs is complex, particularly when optimizing simultaneously for scalable manufacturing processes and navigating the time-consuming and costly iterative cycles of testing and redesigning AAV constructs. In the following blog, we’ll discuss the challenges of AAV therapeutic development and how AI and the “right” data can help accelerate the process.
Quick Review of the AAV Therapeutic Development Process
The AAV therapeutic development process is a meticulous, iterative journey that begins with a strategic construct design, selecting a promoter, transgene, and other essential construct elements. Once the general construct design is established, the next step is to choose an AAV capsid based on the cell or tissue type being targeted, safety profile, and administration route (systemic vs. local).2
From there, a manufacturing platform and process must be selected to produce AAV for pre-clinical studies. A scale-up system for larger-scale AAV production is identified as a program that moves towards clinical testing.
Given the complexity of AAV assembly, the AAV production process is far from perfect. It requires downstream purification and polishing to remove impurities, such as empty or partially filled capsids and contaminating DNA. Finally, QC/lot release assays for viral titers, endotoxins, residual host cell protein contamination, and other critical quality attributes must be developed and validated.
This multi-faceted development process underscores the importance of talent, knowledge, and experience to navigate the intricacies of AAV gene therapeutic development.
Challenges in Each Step of the AAV Therapeutic Development Process
AAV therapeutic manufacturing process itself is intensive; the time and labor cost to generate a single dataset for one construct in a real-world setting is about three weeks and costs $50,000, respectively. However, a gene therapy program rarely investigates a single construct design; many will validate and iterate on 10 or more construct designs.
Specific issues can slow progress as you navigate the AAV development process described above, increasing the cost and time required to validate a construct.
Step 1: Viral Construct Design
Designing an appropriate viral construct for AAV-based gene therapies requires the selection of the right ITR, promoter, transgene, and poly(A) variant for the given therapeutic application. While many of these components are viewed as modular, there can be unpredictable outcomes to assembling these components into an AAV construct. The choices made at this initial design stage can impact production yield, quality, immunogenicity, transduction efficiency, and more downstream.3 Unfortunately, these issues may not be discovered until late in pre-clinical or clinical development, leading to costly redesign and retesting.
Step 2: Plasmid Production and Purification
Once construct design is established, plasmids must be produced at a large scale and high quality. This plasmid acts as input for co-transfection with helper plasmids into adherent or suspension cells, typically HEK293 or HEK293T cells. All plasmids in the transfection protocol must be QCed according to cGMP requirements. Assays confirming the identity, purity, concentration, and other physical and molecular characteristics are done as a part of this process. In addition, the transfection protocols, cell lines, and media used must all be optimized and meet cGMP requirements.
Step 3: AAV Production
Several AAV production systems exist, including mammalian, insect, or viral expression systems. Their transfection methodologies, cell line development requirements, and bioreactor conditions vary. Depending on the AAV-based gene therapy being produced, there may be pros and cons related to the complexity of the process, scalability, yield, and quality of using one methodology over another. For instance, creating stable cell lines for AAV production can be complex, with additional characterization and stability needing to be assessed.
Step 4: Downstream Processing and Characterization
Post-production purification of the AAV-based gene therapy is the final step before formulation and release testing are performed. Ultimately, these downstream purification steps dictate the quality and quantity of the AAV gene therapy product, making them particularly critical.
There is a huge amount of variation and possible approaches to purification related to separating AAV vectors from impurities (cellular and viral), removing empty and other non-therapeutic AAV capsids, and the potency and stability of the purified AAV. This presents a significant challenge for developers to validate a process that works for them and a major cost barrier, particularly for those developers that require significant purification and removal of impurities. To rectify this, there have been attempts to develop standardized downstream purification processes across all AAV-based therapies, yet there has been no regulatory pressure to enforce this.4
Challenges of Getting the ‘Right’ Data for AAV Vector Design
Many of the leaders in the gene therapy field have focused on using AI to solve some of the challenges outlined above. However, for AI to provide valuable insights into these problems, well-annotated, structured, and relevant data for training and testing is required. Given the newness of the gene therapy field, acquiring this data is difficult for various reasons.
Variability in Biological Samples
There remains a lot of methodological and intrinsic biological variability in a living experimental system, which makes much of that data unsuitable for AI. This complication emphasizes proper experimental design and collecting “the right data” for training and testing AI systems. For instance, empty and partially full AAV capsids are common non-therapeutic impurities produced during AAV manufacturing.5 These concentrations can vary significantly depending on construct design and different production parameters.
Data acquisition and data quality issues
Creating effective AI algorithms usually demands extensive data for training and ensuring consistent outcomes. In gene therapy, data availability is often constrained by the infrequency of specific diseases and the substantial expenses linked to data gathering. Moreover, data from diverse sources may exhibit differing formats, quality, and labeling standards. These discrepancies pose challenges in developing precise and dependable deep learning models. Addressing the issue of "data scarcity" involves the deliberate curation of specialized gene therapy datasets strategically tailored for training cutting-edge, niche AI algorithms.
For instance, assessing an AAV preparation's purity, titer, and quality relies on next-generation sequencing (NGS) data. Despite the cost of NGS going down year over year, it is still expensive to do. Moreover, data storage and NGS analysis can become prohibitively expensive when applied to AAV gene therapy development, where more than 10 constructs may be investigated across several different production protocols. In addition, new NGS methods, such as PacBio’s HiFi long-read sequencing, are being developed for AAVs.6 While they have significant advantages for characterizing AAV genomes, integrating the data from these new technologies with legacy short-read sequencing platforms introduces complications.
Strategies for Obtaining the Right Biological Data for AAV Gene Therapy Development
To ensure that variability in data is minimized and data quality remains high, standardization and quality control are necessary for the technologies essential for the gene therapy development pipeline. Lack of standardization in library preparation protocols, sequencing instrument use, and data analysis pipelines are still major complicating factors for NGS.7
Data standardization and quality control measures
In addition, there is still no global agreement on all publicly available data following FAIR guidelines, which would require that all data is Findable, Accessible, Interoperable, and Reusable.8 For AI applications, NGS data should follow FAIR guidelines and, at a minimum, must be properly aligned and annotated with gene-of-interest (GOI), regulatory elements, introns, and other UTRs.
Harmonizing AAV Vector Design, AI and Data to Accelerate Gene Therapy Development
As NGS data and other datasets applicable to gene therapy become increasingly available, AI tools will be improved and accelerate the AAV development process. Form Bio now provides robust in silico capabilities for gene therapy development, spanning from disease models to IND. This diverse set of capabilities positions us as an ideal co-development partner for AAV gene therapy companies, offering comprehensive support throughout the entire drug development lifecycle—from early-stage design to preclinical development and regulatory approval, paving the way for clinical development.
To bolster our expertise, we are strengthening our competitive advantage by acquiring and sequencing what we believe is the most extensive collection of high-quality NGS data on AAV gene therapy constructs.
This AAV gene therapy dataset, generated in collaboration with PacBio, encompasses various genes of interest, promoters, and more. We integrate this valuable data into our training and inference models, enabling us to gain insights into critical aspects such as manufacturing output, design flaws in constructs, impediments posed by secondary and tertiary structures during translation, and the potential for immunogenicity.
AI Disclosure: Feature image was generated by an AI image development tool MidJourney.
Learn more about our AI tools to optimize your AAV vector design today
Schedule Your Discovery CallReferences
- Aldridge, C. How Are AAV Vectors Transforming Gene Therapy? Form Bio. Accessed February 16, 2024.
- Naso MF, Tomkowicz B, Perry WL, Strohl WR. Adeno-Associated Virus (AAV) as a Vector for Gene Therapy. Biodrugs. 2017;31(4):317-334.
- Li C, Samulski RJ. Engineering adeno-associated virus vectors for gene therapy. Nat Rev Genet. 2020;21(4):255-272.
- Grieger JC, Soltys SM, Samulski RJ. Production of Recombinant Adeno-associated Virus Vectors Using Suspension HEK293 Cells and Continuous Harvest of Vector From the Culture Media for GMP FIX and FLT1 Clinical Vector. Mol Ther. 2016;24(2):287-297.
- Aldridge, C. Distinguishing AAV Full/Empty and Fragmented Capsid Ratio. Form Bio. Accessed February 2, 2024.
- Sellami, N. Improving Full-Length AAV Sequencing with PacBio. Form Bio. Accessed February 19, 2024.
- Endrullat C, Glökler J, Franke P, Frohme M. Standardization and quality management in next-generation sequencing. Appl Transl Genomics. 2016;10:2-9.
- FAIR Principles. GO FAIR. Accessed February 20, 2024.
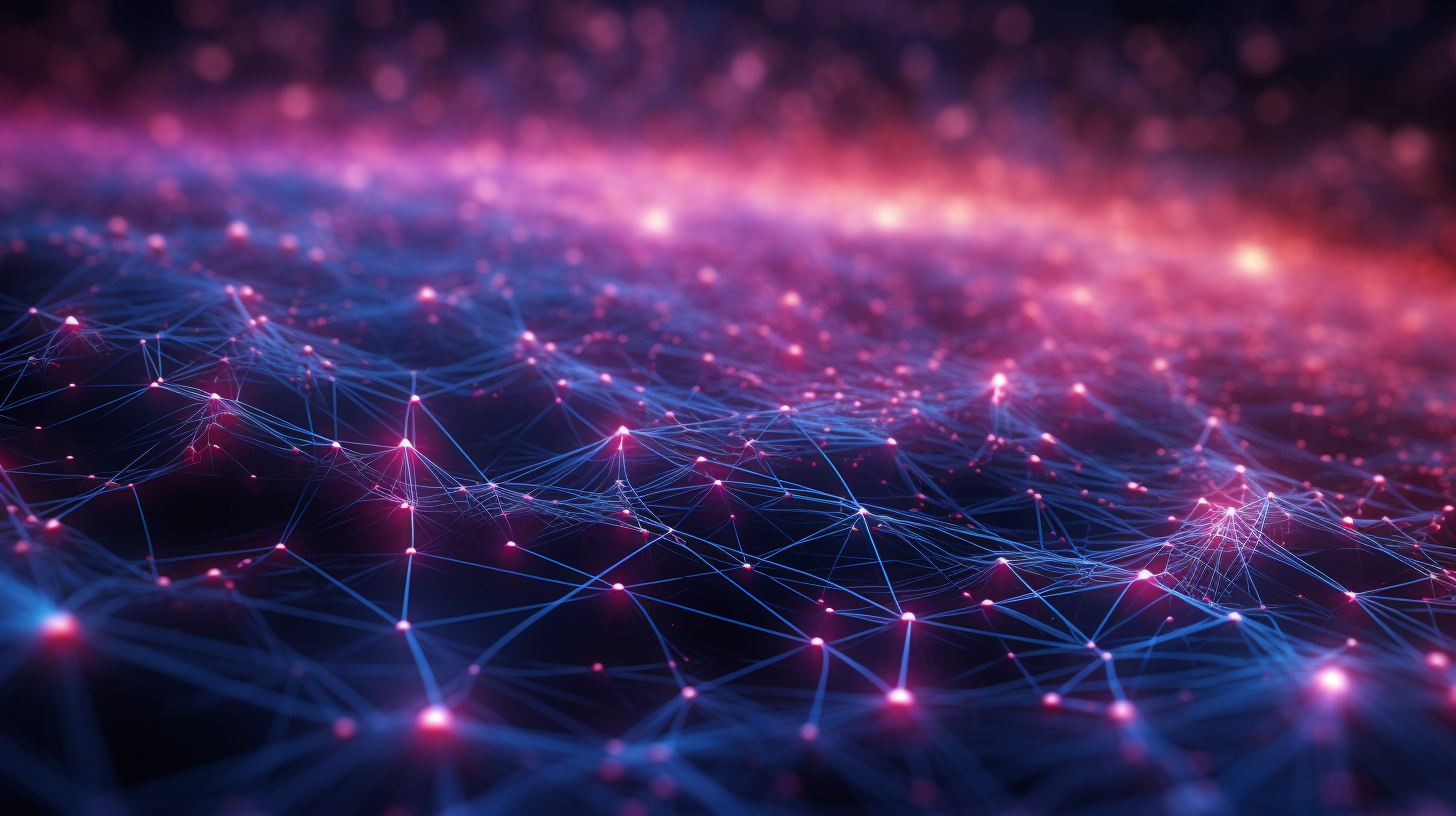