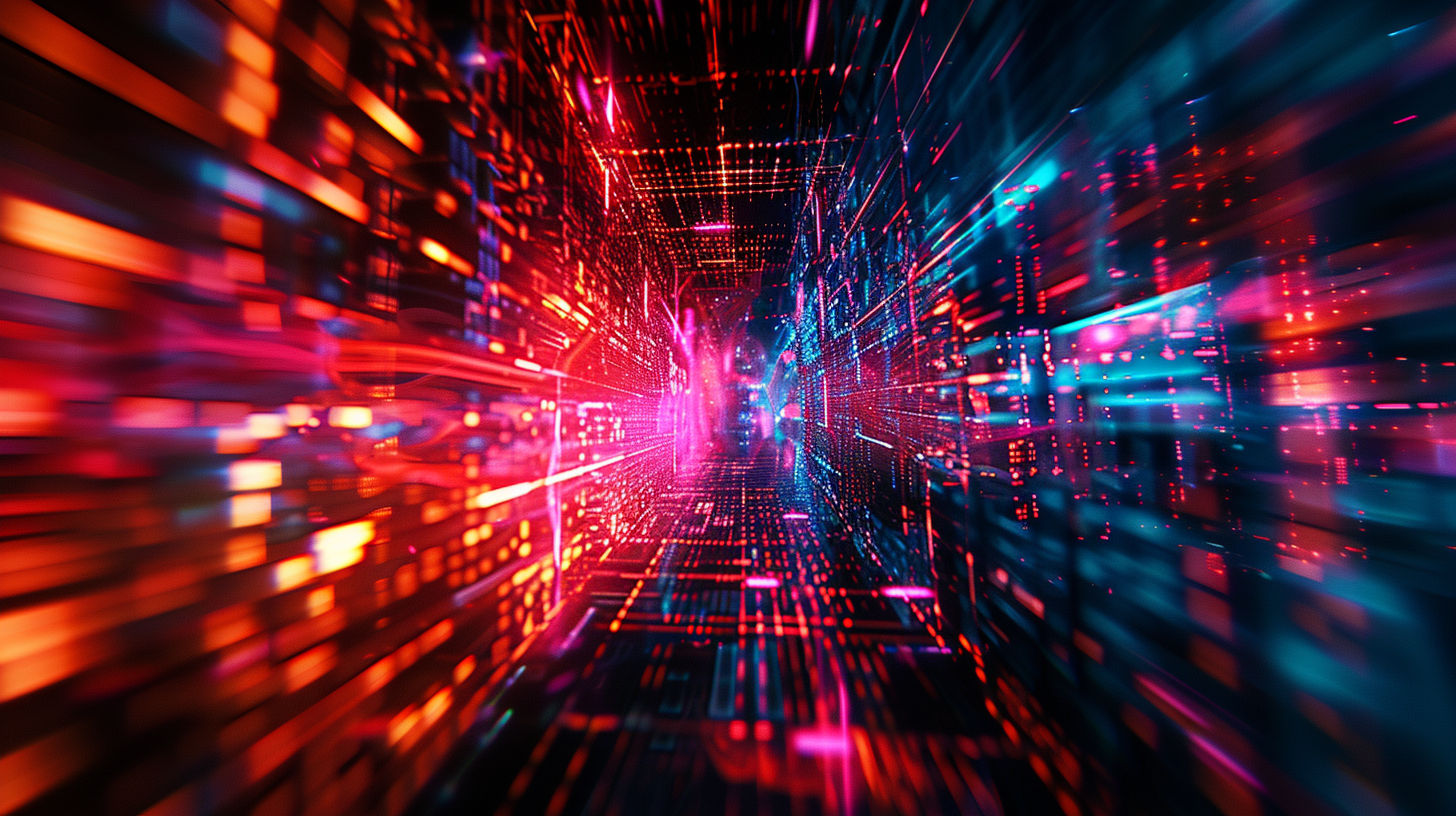
In a wave of progress spanning decades, the journey from Sanger sequencing to simple and sophisticated DNA technologies has revolutionized the accessibility of genetic information, transforming it into a readily available commodity. While genomics holds significant importance in understanding disease etiology, and diagnosing and treating patients, delving into the study of proteins, RNA transcripts, and tissue morphology is just as crucial for advancing human health. Unraveling the interconnected workings of DNA, RNA, protein, and morphology and form linkages between phenotype and genotype, allows both basic and clinical researchers to propel their research, development and diagnostic programs forward.
If data is the new gold, then biological data is like the lost city of gold. Unlocking the value of this data with translational science can be akin to finding El Dorado. Due to the massive amount of data resulting from these assays, capturing the correlations across multiomics data necessitates advanced computational techniques. Recognizing this challenge, Google and Form Bio have partnered to empower researchers in both basic and clinical research realms by facilitating self-service multiomic data analysis workflows and taking their research to new heights.
This initiative provides scientists with greater capacity to focus on engaging in extensive discussions with their collaborations and interdisciplinary teams.
Multiomic Data Analysis and Benchmarking For Precision and Speed
Form Bio’s turn-key workflows simplify complex analytical processes, enabling basic and clinical researchers to effortlessly navigate through multiomic data analysis tasks. With user-friendly interfaces, researchers can easily select desired analyses, configure parameters, and execute workflows with just a few clicks. This streamlined approach enhances efficiency and empowers researchers to focus more on insights and discoveries rather than grappling with technical complexities.
To validate our workflows, Form Bio uses Google Health's test datasets in their GitHub repos. Subsequently, Google Health reviews our processes to ensure alignment with their requirements by employing Illumina’s hap.py for result verification.
The following deep learning workflows are available:
DeepVariant
Genetic variant caller for high throughput sequencer data analysis, including extensions for RNA-Seq analysis1.
DeepSomatic
Employed for analysis of somatic mutations in cancer genomes2.
DeepTrio
Designed for variant calling in families using deep learning, focusing on analyzing the DNA of children and their parents to understand genetic inheritance and mutations3.
DeepConsensus
An alignment-based loss to train a gap-aware transformer–encoder for sequence correction4.
DeepPolisher
Designed to identify errors in genome assemblies5.
AlphaFold
Predicts protein structures with unprecedented accuracy6.
Data Visualization for Multiomic Analysis
In the life science industry, the importance of effective communication of your results to different stakeholders and various ways can not be overstated. The visualization and sharing of data results are crucial for effective communication of complex biological data. Powerful out-of-the-box and ad-hoc data visualization and analysis, without needing to move data around between systems, allows for streamlined collaboration, enhanced data-driven insights, and ultimately the acceleration of scientific discovery.
Form Bio’s expertise in user experience allows life science professionals to create dynamic visualizations that communicate complex biological data effectively in seconds.
The Future of Multiomics Data Analysis
A central question in biology today is “how is the genetic code translated into the variety of traits seen across diverse species”? Using a multi-omics approach, scientists can answer questions about genetic variation and gene regulation which will inform on the processes that contribute to different traits and disease. However integrating and analyzing this data can be challenging. Over the next decade, a key focus area will be integrating biomarker analysis tools into unified processes and dashboards. Better resources and methods for data analysis will be key for amplifying the signal/noise ratio in diverse populations. For example, analysis with pan-genomes should reduce alignment errors that lead to variant artifacts. Developing easy to use tools and dashboards, powered by cloud computing technology will be critical elements in organizing and effectively analyzing these vast and rich datasets, including the application of AI.
By empowering users with easier to use tools for analysis and visualization, with Google-powered multiomics workflows integrated into Form Bio’s user-friendly software, life sciences professionals can efficiently translate data into actionable insights, with increasing precision and fostering accelerated scientific discovery.
AI Disclosure: Feature image was generated by an AI image development tool MidJourney.
Want to make the most of your multiomic data?
Select your free or paid plan today!References
- DeepVariant: Highly Accurate Genomes With Deep Neural Networks. Published Dec 2017. Google Research Blog. Accessed March 2024.\
- Sahraeian, S.M.E., Liu, R., Lau, B. et al. Deep convolutional neural networks for accurate somatic mutation detection. Nat Commun 10, 1041 (2019).
- DeepTrio: Variant Calling in Families Using Deep Learning. bioRxiv. Published April 2021. Accessed March 2024.
- DeepConsensus: Gap-Aware Sequence Transformers for Sequence Correction. bioRxiv April 2021. Accessed March 2024.
- deeppolisher. gitHub. Published Jan. Accessed March 2024.
- Jumper, J., Evans, R., Pritzel, A. et al.Highly accurate protein structure prediction with AlphaFold. Nature 596, 583–589 (2021).
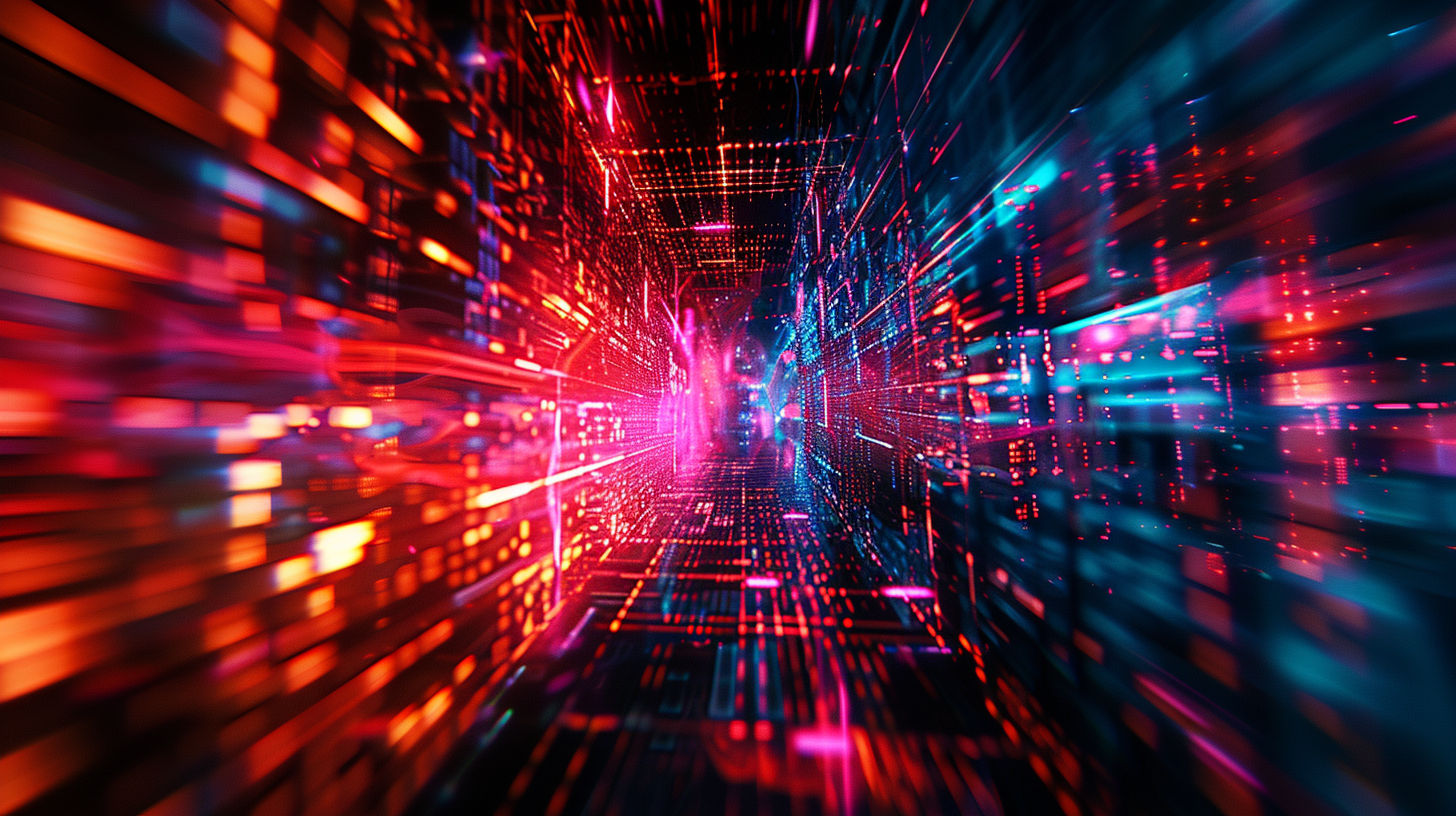