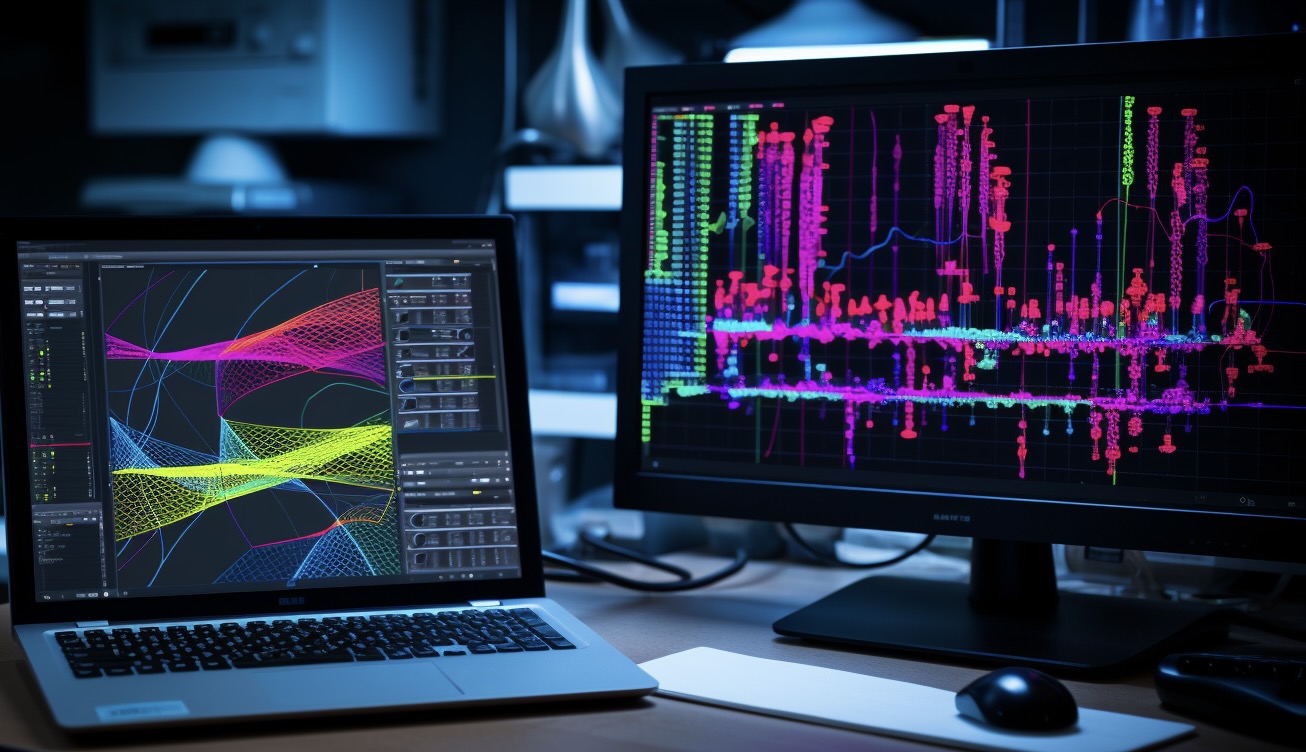
In the current landscape, techbio companies are increasingly forging collaborative partnerships with leading sequencing organizations, cancer centers, pharmaceutical and biotech firms, and instrument manufacturers, with the aim of seamlessly incorporating AI into their operational frameworks. We can now comfortably leverage cutting-edge AI tools to gain profound insights into the intricacies of biology's systems. These insights offer the life science industry unique perspectives into the molecular foundations of diseases, their clinical evolution, and the most efficient methods for treating them with novel or repurposed drugs.
With human clinical trials with AI-designed drugs now ongoing, it's only a matter of time until the FDA approves its first AI-designed drug1. These successes provide a solid foundation for AI’s recent shift into more complex and newer drug development applications, such as monoclonal antibodies and cell and gene therapy2.
As the interconnectedness of the life sciences, AI, and data science continues to evolve, the optimistic vision is that the gap between medical diagnosis and gene therapy treatment will significantly diminish, resulting in speedier and improved outcomes for patients in need.
Implementing AI as a Complementary Scientific Tool
The most effective way to leverage AI in life science research and development is to use it as a complementary scientific tool to harness a scientist's creativity. AI is meant to augment a human decision or action in a way that improves speed, quality or both not replace it3. Moreover, driving the adoption of AI as a complementary partner alongside your data science and wet lab operations will speed up the hypothesis testing process which contains alot of time-consuming trial and error.
Validating In Silico Results with Wet Lab Bench Data
Overcoming scientists' inherent skepticism is a top priority and demands effective communication of AI's benefits to be used to augment scientific process and thinking. Scientists are naturally cautious, preferring concrete data and repeated validations. Therefore, integrating wet lab experimental validation into workstreams is essential.
The process of computational validation encompasses several key steps4. These include aligning the input data with its source, evaluating it against industry standards, conducting back-testing. As for the model itself, it involves scrutinizing its logic, identifying sources of bias, assessing uncertainty, checking for overfitting, and gauging its responsiveness to dynamic changes. Furthermore, the output must be examined to ensure clarity and accuracy.
Practical Tips for AI Implementation in Life Science Programs
Gaining access to data serves as a fundamental requirement for deploying AI solutions in wet lab operations but there are some cultural shifts that need to take place as well. During a recent webinar we hosted with leaders from Nvidia, BCG and ElevateBio, they shared their learnings and practical tips they’ve learned in real world settings that have enabled long term success to reduce operational inefficiencies and cost and enhanced therapeutic end product quality.
Obtain Leadership Buy In
Securing high-level support is crucial. It's essential to help the C-suite grasp the benefits of integrating ai tools to enhance business outcomes and foster a culture aligned with this vision. The core objective is to demonstrate a tangible impact, whether it's in terms of revenue, overall business enhancement, or the broader value you aim to achieve. The key is to illustrate how this impact cascades throughout the organization, a strategy that has consistently proven effective based on my experience.
Start Small, Think Big, & Embrace Failure
The general rule of thumb to handle major change is to start small. Find a good pilot project where you have some data, and gradually scale up your initiatives as you gather valuable insights and refine your approach. This incremental process helps mitigate risks and ensures a smoother transition to larger-scale implementations. It's also crucial to perceive setbacks as opportunities to learn and encourage an entrepreneurial mindset.
Define Where Best to Apply AI Tools and Prioritize
A critical component of integrating AI is to possess a deep understanding of scientist workflows. This insight is essential in identifying the tasks most suitable for AI augmentation. The best way to understand where to apply AI tools requires collaboration between technical and biological expertise to tackle the right kinds of problems. Start by breaking down the user experience into specific tasks. Then prioritize tasks from most commonly used to least commonly used. From there you can evaluate the most common tasks to understand if they would be amenable to AI assistance.
Create Cultural Alignment and Cross-Functional Governance
Ultimately, the goal is to ensure AI projects not only receive approval but also see widespread adoption. Identifying advocates is pivotal. These individuals are usually the frontline champions who can drive the implementation forward. It’s essential to create a cultural shift and emphasize that AI is meant to augment a human decision not replace it.
Recognizing that we're all on the same team is essential, whether you're a scientist well-versed in manufacturing chemistry or a data scientist or AI engineer. It's crucial to approach these interactions with an open mind, as this soft skill facilitates bridging the gap.
Leveraging AI in Gene Therapy Pre-Clinical Development Programs
Form Bio is the leading AI powered gene therapy platform that combines data, technology and expertise in silico solutions that reduce time to clinic while increasing the yield and the safety in a secure environment. We work collaboratively with our strategic partners to design and nominate a gene therapy candidate(s) for clinical development whereby multiple constructs are designed and tested with a final candidate moving into clinical trials.
AI Disclosure: Feature image was generated by an AI image tool MidJourney.
Want to learn more about our gene therapy AI technology?
Schedule your discovery callReferences
- First Human Trials Begin for AI-Drug Designed Drug. The Motley Fool. Published June 26, 2023. Accessed October 15, 2023.
- Cesaro, A., de la Fuente-Nunez, C. Antibiotic identified by AI. Nat Chem Biol (2023).
- The economic potential of generative AI. McKinsey & Company. Published June 2023. Accessed October 2023.
- Elliot A. Validating Computation Models with Real World Biological Experiments. Form Bio Resource Center. Published August 2023. Accessed October 2023.
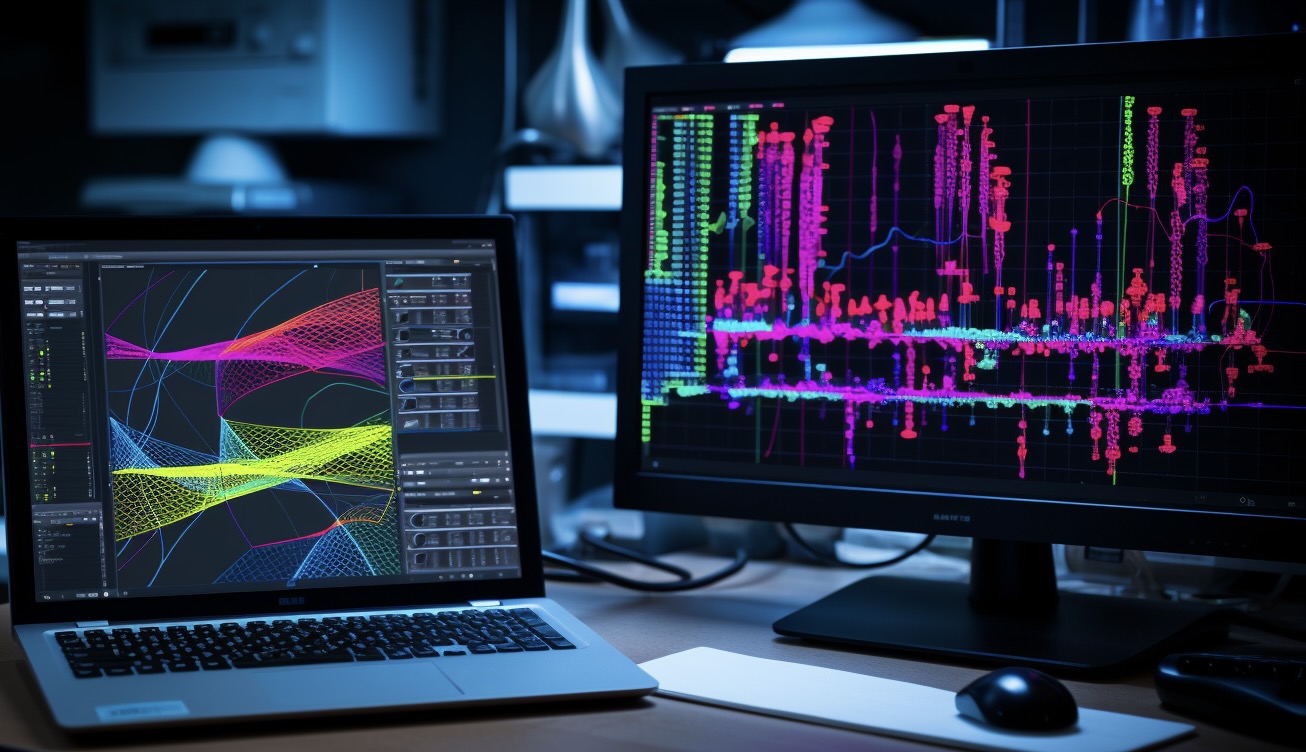