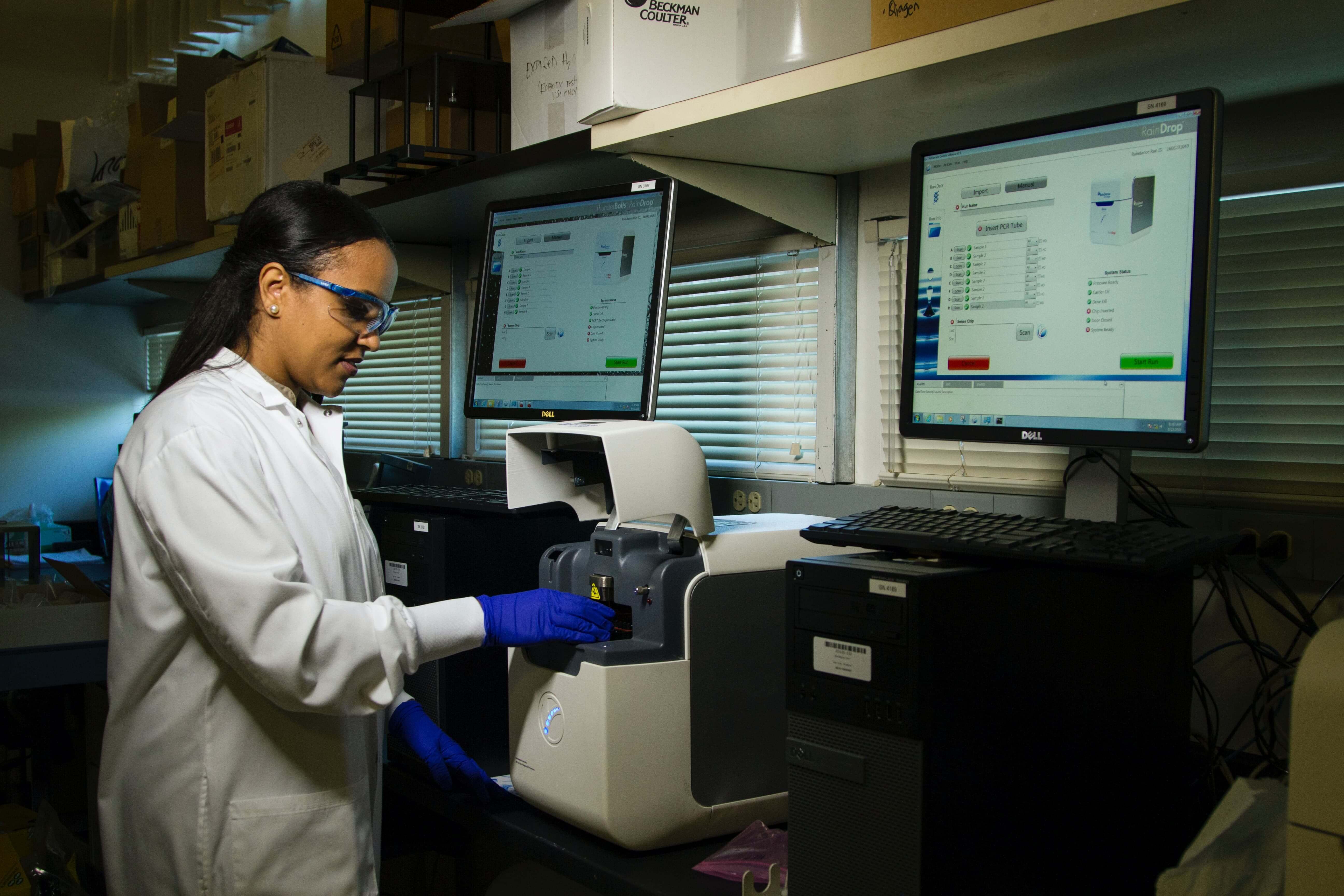
Sequencing technologies have come a long way since the days of the slab gels - a process that took several days from beginning to end and yielded 300 base pairs per run - on a good day. What used to be a largely manual process has long become fully automated and next generation sequencing technologies are now capable of sequencing whole genomes in hours. Prices have also rapidly declined, the newest system by Illumina promises to sequence a human genome for just $200 1 - a far cry from the 300 million dollars the first one cost back in the early 2000s.
But new and innovative sequencing technologies are not yet maxed out, new, faster and less expensive technologies will emerge and with them new applications become possible that even a few years ago seemed ambitious.
Next generation sequencing has been dominated by Illumina which has controlled 80% of the global sequencing market. However, over the next couple of years key patents that helped the company secure their market position are expiring paving the way for more competition. The top 10 players in the sequencing market are Thermo Fisher Scientific, Agilent, Rio-Rad, Qiagen, PerkinElmer, Roche, and BGI Group.
Third generation sequencing is less established, the main companies offering instrumentation, consumables and/or services are Pacific Biosceinces and Oxford Nanopore Technologies with a number of young start-ups like Ultima Genomics that announced $100 full genome sequencing this year.3 Other up and coming sequencing companies include Singular Genomics, Element Biosciences, MGI, and others.
Join us on a short tour to explore how sequencing evolved and how scientists make sense of big genomic data.
What Are the Different Types of Sequencing Technology?
Sequencing technologies are generally categorized as first-generation, next generation sequencing (NGS) and, a recent addition, third generation sequencing.
First-gen sequencing used various approaches to read fairly short DNA fragments at low through-put and high cost. Using these techniques severely limited the applications, e.g. to sequencing simple genomes such as viruses and bacteria or parts of human genes or regulatory regions of genes.
Development of next generation sequencing was driven by the need to increase the amount of DNA that can be sequenced. Different techniques were developed which are all capable of massively parallel processing by sequencing millions of DNA strands simultaneously.
This enables scientists to sequence whole genomes, research genome diversity, perform metagenomics studies, sequence RNA for comprehensive gene-expression profiling studies – to name but some of the many applications.4
However, all NGS approaches face common challenges 5 such as:
- small read lengths (<300 bp), which create difficulties in de novo assembly
- hard to sequence regions, e.g. those with high/low GC content or tandem repeat regions
- fragmentation that makes de novo genome assemblies difficult and can lead to entire portions of the genome or vital genes missing.
Third generation approaches take sequencing to a whole new level and address these issues. The two fundamental features that distinguish NGS from third gen sequencing are the ability to sequence long reads of single DNA and RNA molecules without the need for PCR amplification as well as the ability to perform real-time analysis of the produced data. 6,7 In addition, they allow sequencing of regions of genomes that previously were difficult to sequence correctly or yielded biased results, e.g. due to their GC content.
Third gen sequencing techniques simplify sequencing of whole genomes from human, animal, plant and microbial genomes where the long reads with greater overlaps facilitate the assembly process, help with mutational analysis, and identification of new SNPs. RNA sequencing enables the detection of RNA modifications and novel miRNAs, among many other applications.
In terms of applications, sequencing technologies are currently used for whole-genome sequencing, epigenetic, metagenomics, identification of non-coding RNAs and protein binding sites, and gene expression profiling using RNA sequencing.
These technical innovations have led to impressive growth rates. According to analyst reports, the sequencing market will growth by over 20% annually to reach a market size just shy of $24 billion in 2026. 8
As sequencing techniques have evolved, so have the computational models needed to make sense of the big data these methods generate.
In the next section we discuss the computational models and approaches used for both NGS and TGS.
Computational Challenges in Next Generation Sequencing
NGS, sometimes also known as short-read sequencing, requires the use of specialized bioinformatics tools and complicated post-processing pipelines.
Typically, NGS bioinformatics workflows are divided into three analysis steps:
- Primary: detection Signal analysis, base calling s wella s steps such as base quality scoring and demultiplexing
- Secondary: alignment of the reads against a reference genome or de novo assembly, if no reference is available
- Tertiary: variant annotation and filtering as well as prioritization, data visualization and reporting
These steps represent major challenges, especially puzzling the short, overlapping reads together into a continuous sequence, which is made even more difficult if a sequence contains repetitive elements. Alignment of the new sequence with existing sequences that are generally obtained from databases create further computational challenges, e.g. misalignment issues if there is a discrepancy between the new sequence and the reference and determining when a discrepancy should be considered a real variation or just a misalignment.9
Overcoming Computational Challenges in Third Generation Sequencing
Third generation sequencing with its long reads makes assembling genomic sequences more straight-forward. The major bioinformatics challenge of TGS is the high sequencing error rate that requires new alignment and error correction algorithms as well as challenges around interpreting complicated structural variants.10
Another computational challenge has to do with the large amount of data that is generated by TGS approaches and that needs to be aligned or de novo assembled.
Over all, development of more sophisticated computational tools along with advancements in computing power have been major drivers of the global demand for sequencing.
Conclusion
Sequencing has seen many remarkable innovations over the last decades that have increased the speed and reduced the cost by many orders of magnitude and enabled application of these technologies in a broad array of disciplines, from basic to medical research, from drug development to diagnostics and clinical applications. Sequencing is also used in agriculture, e.g. to understand microbial communities in the environment.
Many of the remarkable medical advances we have seen over the last years would not have been possible without increasingly fast and cheaper sequencing technologies as well as the computational tools that allow researchers to bring order to apparent chaos and make sense of seemingly random strings of nucleotides.
The future for sequencing looks bright, third generation sequencing approaches make sequencing feasible for the individual and allows to analyze not just DNA but importantly RNA on a single cell basis – the next important step to understanding the biology of health and disease on a granular level.
Interested in learning more about how Form helps you make sense of sequencing data?
Get Your DemoReferences
- Illumina Aims to Push Genetics Beyond the Lab With $200 Genome. Bloomberg. Published September 29, 2022. Accessed October 30, 2022.
- International Human Genome Sequencing Consortium Publishes Sequence and Analysis of the Human Genome. National Human Genome Research Project. Published February 12, 2001. Accessed October 30, 2022.
- Ultima Genomics Claims $100 Full genome Sequencing After Stealth $600 M Raise. TechCrunch. Published May 31, 2022. Accessed October 30, 2022.
- Gupta N et al. Next-Generation Sequencing and Its Application: Empowering in Public Health Beyond Reality. Microbial Technology for the Welfare of Society. Pages 313–341
- Wee Y. The bioinformatics tools for the genome assembly and analysis based on third-generation sequencing. Briefings in Functional Genomics. 18 (1): 1–12. (2019).
- Athanasopoulou K. Third-Generation Sequencing: The Spearhead towards the Radical Transformation of Modern Genomics. Life (Basel). 12(1): 30. (2022).
- Long J. Third-generation sequencing: A novel tool detects complex variants in the α-thalassemia gene. Gene 822. (2022).
- DNA Sequencing Market Size to Rise by USD 23.56 bn | 38% of the market growth to originate from North America| Technavio.PR Newswire: Published May 16, 2022. Accessed October 11, 2022.
- Pereira R. Bioinformatics and Computational Tools for Next-Generation Sequencing Analysis in Clinical Genetics. J Clin Med. 9(1): 132. (2020).
- Tiantian X. The third generation sequencing: the advanced approach to genetic diseases. Transl Pediatr. 9(2): 163–173 (2020).
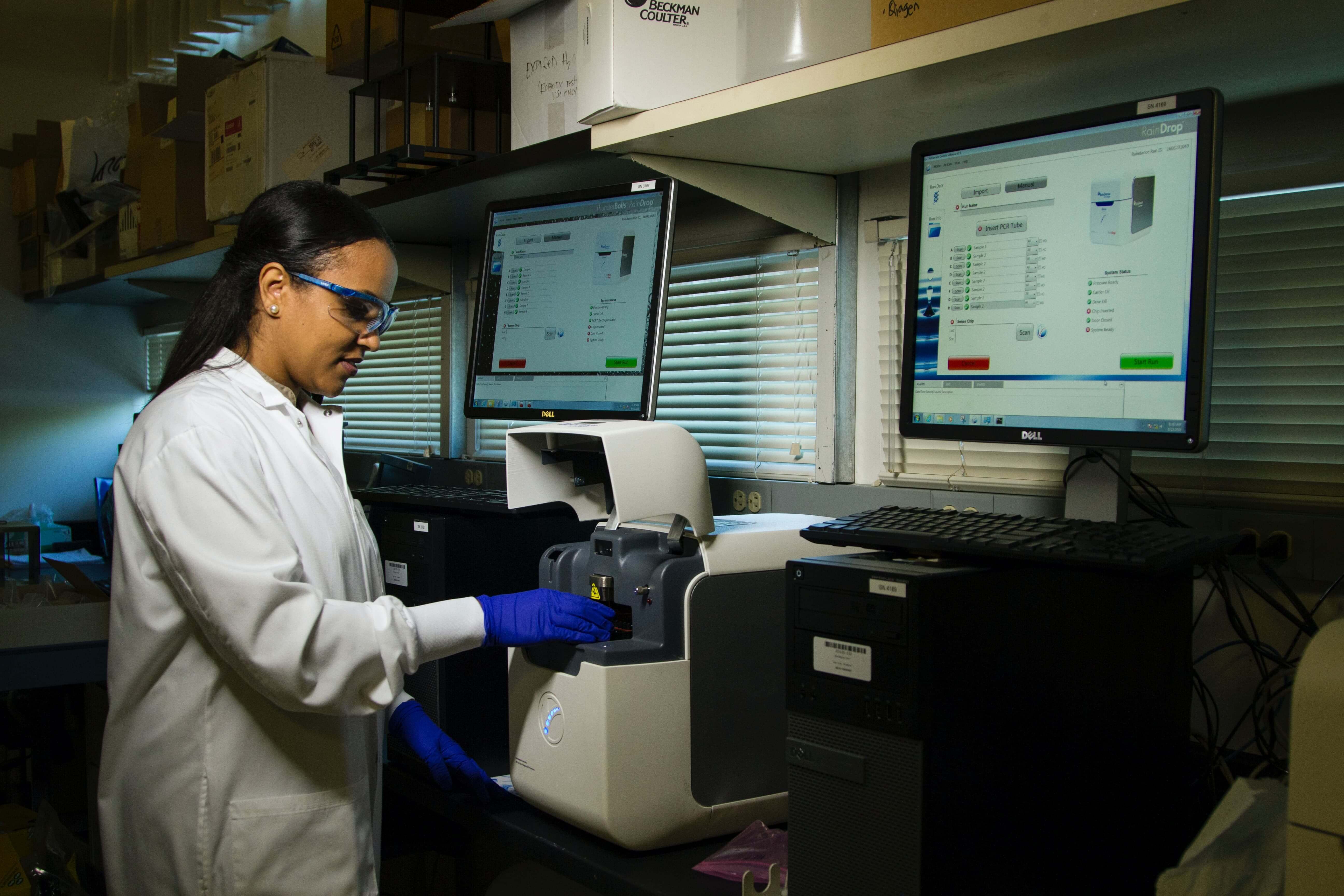