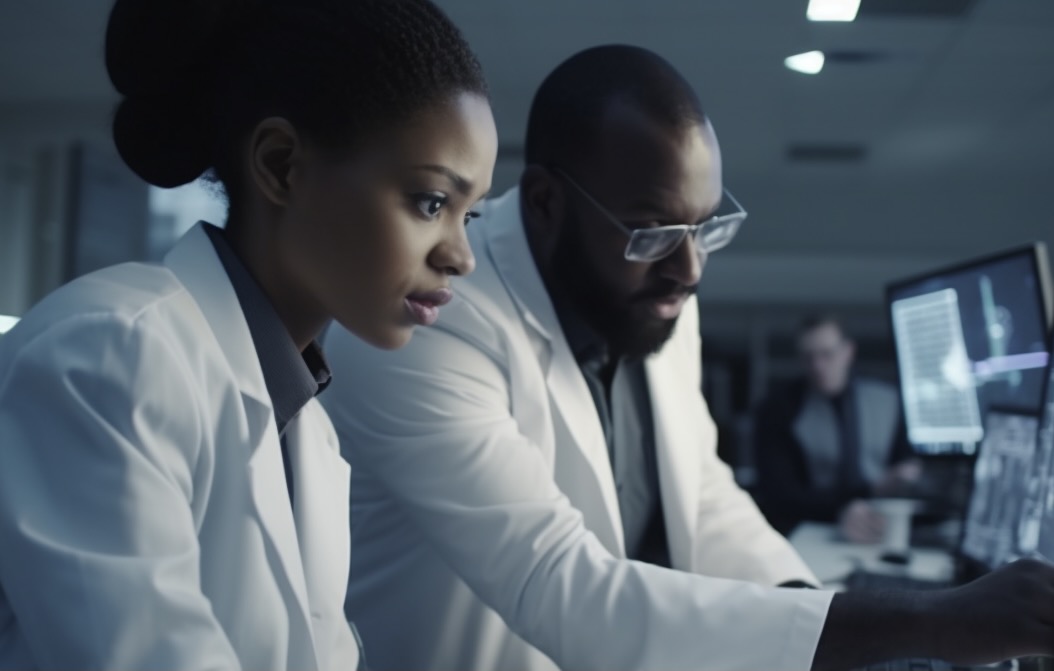
The expedition of artificial intelligence (AI) into the biomedical space has been facilitated by the rapid push to digitize “wet lab” research and the explosion of data from multiomics experiments. Furthermore, microscopy and other imaging data present huge opportunities for applying data science and machine learning models toward a robust suite of high-performance computational tools.
Such tools promise to improve all of the life sciences from basic research (understanding the living world) to translational research (applying basic research outputs to clinical situations), and actualization to clinical practice toward the improvement of human health and wellness. As cell and gene therapies ascend for the one-shot curing of life-threatening genetic diseases, there is an excellent opportunity to merge the in silico and in vitro world with a common goal of developing safe, more effective, and highly manufacturable therapeutics.
Now, the recent hype around AI and the bold promises already made in healthcare and life sciences has raised appropriate skepticism and critical awareness. Questions around the ethics of health data collection and usage, bias in machine and deep learning models, and a lack of explainability are all contributing to a wide distribution from fear to mild aversion when it comes to adopting AI products in the biomedical space. In this blog post, we’ll explore the development of algorithms and demonstrate the value of wet-lab validated AI tools for extending biological research into the digital age and accelerating that research toward equitable advances in human health.
The Importance of AI in Leveraging the Potential of Biological Data
Life sciences research (basic, translational, and clinical) produces a vast amount of biological information, but extracting useful insights from this collection of multimodal data remains a major challenge. In addition to “big data” technologies like multiomics, imaging (microscopy, MRI, etc.); there is a plurality of underutilized data hidden in analog sources, poor formatting, or both. Information recorded in paper notebooks including detailed protocols, experimental notes, and observations are potentially rich sources of metadata, features, and labels that could be harnessed to uplevel the value of experimental results. Finally, digitizing the formal academic literature, which goes back to books and scientific letters in the 1500s, is still underway.1 Less formal scientific writings are dated to ancient Mesopotamia, to give a full view of how much information there may be in total.
To take advantage of the island of misfit data, we need to build a symbiotic relationship between biomedical researchers, data engineers, and AI scientists. A data-centric approach to experimentation that includes data integration, statistical analyses, and downstream analysis in the hypothesis generation and experimental design is required to push this intersection of fields forward. AI tools can be major accelerators to such data-centric methods. As an example, Boiko et al. recently utilized transformer-based large language models to analyze published scientific literature and design a chemical synthesis protocol.2 This protocol was then executed using automated instruments, from which the collected data was then ready for further analysis and retraining the models for the next synthesis experimental design. This looped approach to data collection, model development, and model testing demonstrates a strategy toward experimental design that falls a bit outside the traditional “scientific method” but lends itself to a more holistic approach for biomedical research.
While harnessing the combined strengths of biological systems, data science, and AI has enormous potential to augment wet lab research, it should be accompanied bywet lab research. This allows for iteration on question-data-math/model fit, an important process for ensuring that the right data is collected for the question being asked and the most appropriate mathematics or AI model is trained on that data in pursuit of the right results to address the question. Substituting wet lab research is neither feasible, nor desired. It is the combined strengths of these approaches, when properly utilized, that are showing promise for better basic research design, expedited translational research, and predicted outcomes from clinical research.
Considerations for Using AI with Biological Data
After determining that the question being asked is appropriate for an AI-based solution, there are several factors that warrant consideration when applying AI in the life sciences. They fall into broad categories including “ethical AI,”,data, modeling, and validation, which are highlighted below with examples of some of the questions that should be asked. In contrast to a non-healthcare application, the “validation” category here must also include the wet lab or clinical validation. By actively tackling these challenges, researchers can enhance the reliability, fairness, and overall safety of AI systems.
Ethical AI
There are a lot of opinions and definitions floating around for what ethical AI might look like.3–6 There is general agreement that any ethical system for AI must include definitions and guidelines for bias, fairness, trust, transparency, privacy, security, and responsibility. There are open calls for additional guidance on topics like interpretability, governability, sustainability and more. Furthermore, there are globally diverse approaches to the increasing use of AI in general and the convergence of different perspectives is perhaps still a question of ‘if’ rather than ‘when.’
Biological Data Source and Other Data Issues
The old adage is “garbage in = garbage out.” It describes one of the main considerations for using AI in the life sciences:, The data source and other important data issues include processing, persistence, storage, privacy, security, and safety are necessary to fully understand in order to trust your AI models. Below are specific questions to ask.
Data Source
- How much data is needed vs available?
- How was the data generated/collected?
- How diverse is the dataset?
Data Processing
- What transformations or structuring steps were done in preparation for AI (aggregation, etc)?
- If the data were labeled for use with AI, then how?
- Was the data validated for use with AI (missing values, etc), and how?
Data Persistence and Storage
- Where is the data stored and in what format?
- How and when is data cached and deleted?
- How scalable is the data storage system?
Data Privacy, Safety, and Security
- How are the data redacted or de-identified to protect any personally-identifiable information?
- How are the de-identified data being secured from reconstruction?
- What are the access controls for users, AI models, and 3rd party software?
Choosing the Right Model
Choosing the right model for the question being asked is the next crucial step in applying AI to healthcare and life sciences right after determining that AI is the way to go rather than a standard mathematical framework. Not all problems have a good AI solution. Apart from the data itself and the question being asked, some of the critical considerations for the model include: algorithmic bias, transparency, generalizability, reproducibility, regulation, and deployment.
Computational Validation
Computational validation includes reconciling the input data with the source, measuring it against industry benchmarks, and back-testing; for the model itself, inspecting the logic, sources of bias, uncertainty and overfitting testing, and response to dynamic changes.7,8 The output should also be investigated for clarity and accuracy. Apart from the training and testing datasets, an evaluation or validation dataset or partition is needed, as it allows the hyperparameter tuning to be done iteratively and independently from testing and training. These validation steps are well-established though unevenly implemented. Less well defined, but increasingly acknowledged as important, is the concept of wet lab or clinical validation for AI models in healthcare and life sciences.
Wet lab validation and clinical validation means testing a prediction or result from an AI tool in the relevant biological system or with unseen clinical data (not used for testing). This often requires collaborations and partnerships to get the right expertise, equipment, etc. on the same project. Some critical considerations for wet lab validation include experimental design, heterogeneity of samples/data, and robustness of the data collection paradigm (sequencing machine, MRI, etc).A recent noteworthy example by Moor et al. showcases the creation of a comprehensive medical AI model trained on diverse datasets.9 Academic labs are increasingly incorporating AI into their research to accelerate discovery and they are well positioned to test the novel hypotheses and predictions from their AI models.10,11 This breakthrough has the potential to significantly improve the accuracy and efficiency of healthcare. The increasing number of successful applications underscores that AI can revolutionize various domains of the life sciences, including basic research, drug development, and diagnostics. Having established that we can use AI tools for a variety of applications in healthcare and life sciences, what is left are the questions of how and should we?
Trust is a major issue with AI adoption particularly in healthcare and life sciences. All of the excitement around LLMs with the launch of ChatGPT was tempered reasonably quickly by the “hallucinations” and major errors.12 Experiencing those types of issues - and many others - with personal health data, in particular, decreases trust and slows adoption. The importance of thoroughly validating AI models cannot be understated.
How Form Bio is Using AI with Wet Lab Data to Advance Gene Therapy Development
At Form Bio, our goal is to utilize our proprietary AI algorithms to identify gene-disease associations, accelerate the discovery of gene-editing techniques, and drive biomanufacturing advancements, among others.
With our newly launched product, FORMsightAI, you can utilize a novel AI-based framework can effectively decrease time and costs linked to the biomanufacturing of AAV vectors, which are pivotal for delivering potent gene therapies. By integrating FormSightAI early in preclinical research, gene therapy developers can proactively anticipate construct problems and generate more “manufacturable” vectors, before these problems manifest in the later stages of clinical testing.
The models we developed for FORMsightAI are undergoing a thorough wet lab validation with several partners. We are using these experiments to tackle several questions discussed in this blog post including but not limited to:
- How reproducible and robust is our training data?
- How well do our models generalize to unseen data?
- How accurately do our model predictions match observed biological results (for example, viral titre and construct issues like truncations)?
- What additional data can we collect to improve the model confidence intervals?
The integration of AI predictions in biological research (basic, translational, and clinical) is an exciting and promising field that is still in its early stages but progressing rapidly. Through collaborative efforts among researchers, AI experts, and wet lab scientists, we are paving the way for an innovative AI-enabled approach to a personalized healthcare system and accelerated discovery. This emerging technology holds the potential to revolutionize the way we understand and treat diseases, providing new insights and opportunities for targeted interventions. With further advancements and continued collaboration, we can unlock the full potential of AI in improving patient care and outcomes.
AI Disclosure: Feature image was generated by an AI image tool MidJourney.
Want to stay up-to-date with gene therapy R&D trends?
Sign up for our newsletterReferences
- Early Modern Science Collection | Harvard Library. Accessed August 2, 2023.
- Boiko DA, MacKnight R, Gomes G. Emergent autonomous scientific research capabilities of large language models. Published online April 11, 2023. Accessed July 10, 2023.
- Smith B. How do we best govern AI? Microsoft On the Issues. Published May 25, 2023. Accessed August 2, 2023.
- Google AI Principles. Google AI. Accessed August 2, 2023.
- US Department of Defense Adopts Ethical Principles of Artificial Intelligence. Published February 2020. Access August 4, 2023.
- US Department of Health and Human Services. Trustworthy AI (TAI) Playbook. Executive Summary. Published September 2021. Accessed August 4, 2023.
- Lotfollahi M, Rybakov S, Hrovatin K, et al. Biologically informed deep learning to query gene programs in single-cell atlases. Nat Cell Biol. 2023;25(2):337-350.
- Tsopra R, Fernandez X, Luchinat C, et al. A framework for validating AI in precision medicine: considerations from the European ITFoC consortium. BMC Med Inform Decis Mak. 2021;21(1):274.
- Moor M, Banerjee O, Abad ZSH, et al. Foundation models for generalist medical artificial intelligence. Nature. 2023;616(7956):259-265.
- Walsh I, Fishman D, Garcia-Gasulla D, et al. DOME: recommendations for supervised machine learning validation in biology. Nat Methods. 2021;18(10):1122-1127.
- Hosny A, Bitterman DS, Guthier CV, et al. Clinical validation of deep learning algorithms for radiotherapy targeting of non-small-cell lung cancer: an observational study. Lancet Digit Health. 2022;4(9):e657-e666.
- Alkaissi H, McFarlane SI. Artificial Hallucinations in ChatGPT: Implications in Scientific Writing. Cureus. 15(2):e35179.
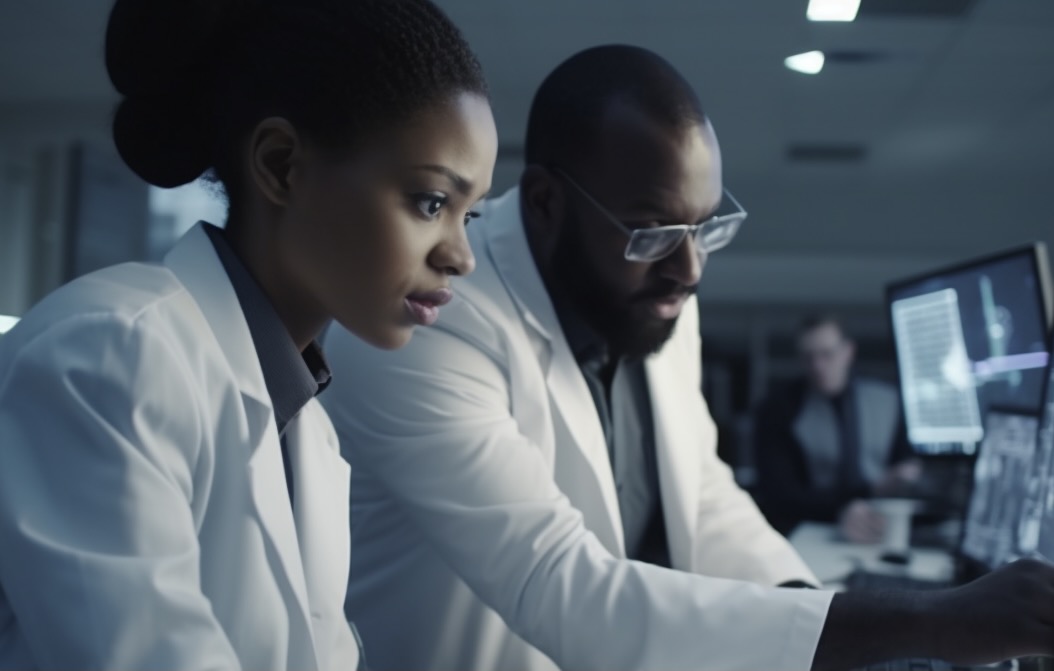